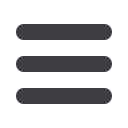
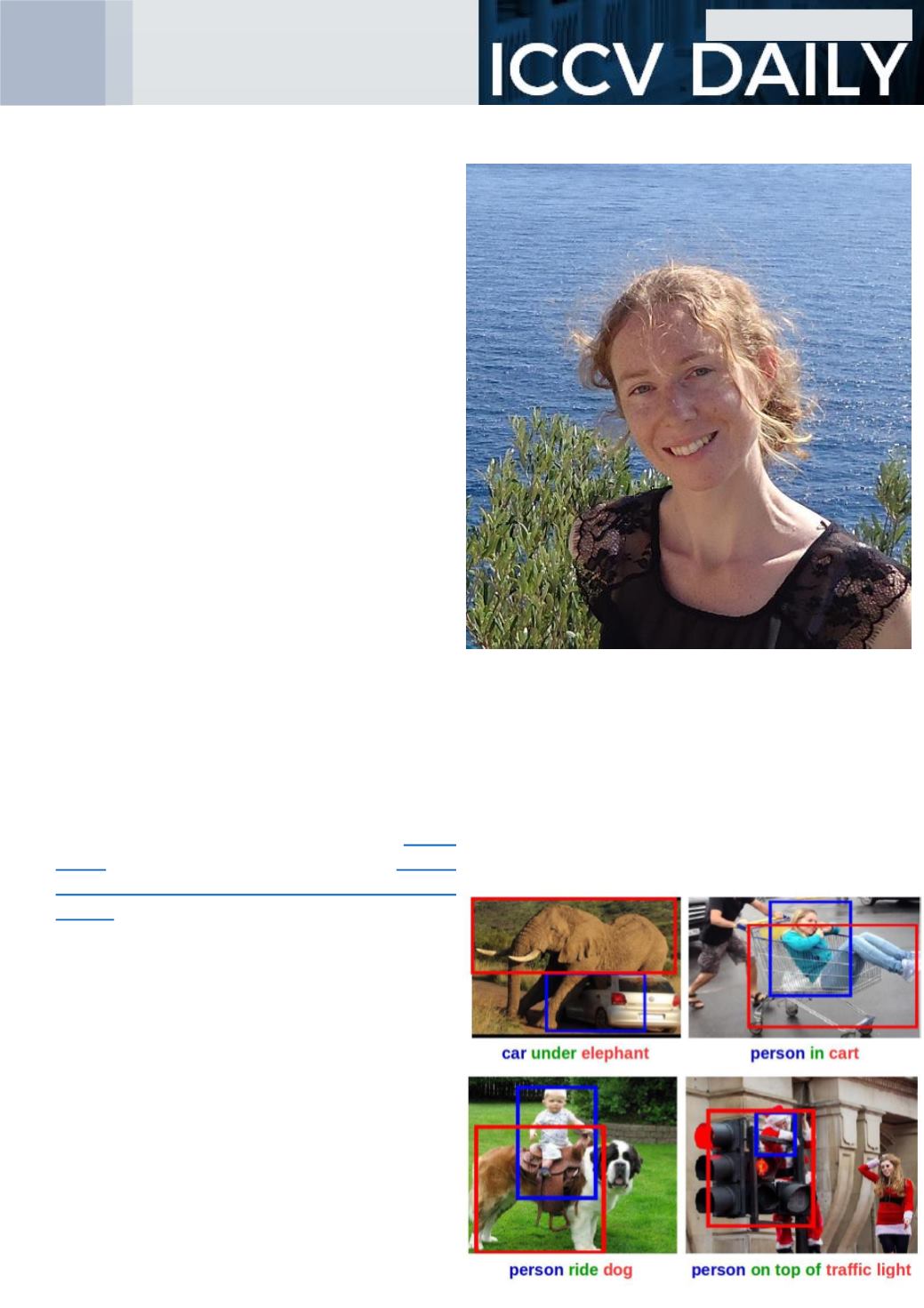
To begin, we ask Julia about working
with
Cordelia
: “
It is very nice. She is a
very efficient person. Nothing is
random: she goes straight to the point.
We are never losing time
.”
This work is called
Weakly-Supervised
Learning of Visual Relations
and its
goal is to learn relations between
objects in images, using only weak
supervision for the relation. Typically,
the input of this method at training
time will be an image with image-level
triplets of the form: subject, predicate,
object. For example, there is a person
riding a horse in an image, but they do
not know the localisation of the
objects. They will train the method to
learn a classifier for the predicate –
riding, in this example – using only this
kind of supervision.
This task was first introduced at ECCV 2016 in a paper called Visual Relationship Detection with Language Priors , by Cewu Lu , Ranjay Krishna ,Michael Bernstein
and
Fei-Fei Li
. That
paper solved the task described above,
to detect the object in a certain
relation in images; however, at the time
of publication, it was not addressed
with weak supervision. That is the
novelty of this work.
The development came about because
the team had been interested in
relations between objects for Julia’s
thesis. In their lab at
Inria
, they had
been working with weak supervision,
so it was natural to think about doing
this task with weak supervision. Julia
adds that it is important to do this with
weak supervision because it is a very
challenging problem to get annotations
at box-level for the relations.
Julia explains: “
If you take natural
images you will have a lot of objects in
these images and the objects will have
many different interactions. If you want
8
Friday
Julia Peyre is a PhD student at
Inria Paris, supervised by Josef
Sivic, Ivan Laptev and Cordelia
Schmid. She speaks to us about
her upcoming oral and poster.
Julia Peyre
Weakly-Supervised Learning of Visual Relations