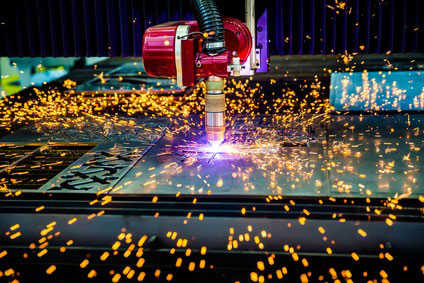
Use of sensors to monitor machinery operations
In many cases, sensors are an integral part of the product (such as with CPU), whereas in other cases sensors are mounted afterwards either to get external reading or to inspect the final product. For the latter case, computer vision and machine learning techniques are particularly useful in studying features of the machine, part, or product based on sensory information. Sensory readings are used to tailor sophisticated algorithms, acting as an alert system, allowing timely reaction in response to possible propensity to malfunction, adjust machine parameters accordingly, or stop machine operation altogether. The reasons for failure or characteristics of the fault, can be studied in the aftermath. The recorded data can further be used to study the reason for failure and to construct a database for fault classification used in machine learning methodologies.
Normal operations vs machine fault detection
The expected reproducibility in manufacture makes the use of visual pattern recognition especially attractive. In this sense, visual patterns are used to detect any deviation from the predefined normal physical characteristics of the manufactured part. A physical pattern can be considered as a map (template or plan) of a PCB, length, width, volume, or any combination of each. However, the definition of pattern can be extended beyond the physical sense and point to a sequence of operations, or combination of sensory information. For example, the study of ‘normal’ machine operation based on integration of vibrational and thermal information can be thought of a template pattern. To create such a template, the redundancy in the vibrational modes signature needs first to be reduced by methods such as principal component analysis, in order to generate only the most discriminative frequencies. In the feature space, the normal operation characterized by a set of frequencies and a thermal signature generates a pattern with soft borders. That is, the range of ‘normal operation’ can thus be studied.
Once a template has been constructed, the outputs of sensors are periodically sampled and tested against the template. The measure of deviation from patterns of normality should depend on the properties of the machine’s operation. For example, patterns of operations that might lead to failure can in some cases be determined over a long time, like the ticking sound of a hard drive, which can be heard weeks before an actual crash occurs; in other cases it remains a particular temporary event. For this reason, studying the pattern leading to a failure is of crucial importance.
Machine learning to analyze failure patterns
Both machine learning techniques and statistical failure analysis are utilized to study failure patterns. Once feature have been collected over time and failures have been recognized, a machine classifier can be used to give an indication of the (instantaneous) hazard of failure. On the other hand, reliability analysis techniques hold a repertoire of methods to predict failure rates and probabilities based on the collected sensory data. For example, competing risks models and proportional hazard models can give rise to a statistical analysis providing an indication of the risk of failure based on the weights of the prognostic factors (feature values).
Classification of faults after their detection is another aspect bearing economical interest for manufacturers. For this end, classifiers are widely utilized but require supervised learning procedures, which might not always be feasible when the nature of the fault is unknown. It is therefore useful to perform non-supervised pattern extraction of the signal of sensory features to be able to dynamically cluster feature patterns and distinguish faults into their own groups. Many times, non-supervised clustering can shed light on the initial reason causing the fault.
The combination of statistical modeling with feature extraction, machine learning and pattern recognition in fault detection has been proven to reduce production costs and product loss, as well as increase the quality of the yield. The economic benefits of constructing a fault detection and classification system based on sensors is great due to the relative low cost of hardware and the availability of advanced computer vision machine learning techniques to interpret generated data. RSIP Vision promotes the integration of advanced machine learning techniques with high quality statistical analysis to construct high quality algorithms for our clients‘ applications. To learn more about RSIP Vision’s operations around the globe, please visit our project page.