Intracranial hemorrhage is defined as bleeding within the cranium. Bleeding can occur within the brain parenchyma itself, giving way to what is called intra-axial bleed or intracerebral hemorrhage. On the other hand, bleeding outside the brain tissue is referred to as extra-axial bleed. Subtypes of extra axial bleed include epidural, subdural and subarachnoid hemorrhages.
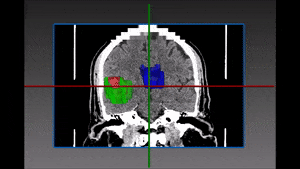
The intracranial hemorrhage might cause a Phenomenon called “mass effect”, meaning that the hemorrhage causes areas of brain tissue or brain structures surrounding it to be compressed and injured, due to elevated pressure in the restricted space within the skull. This could result, together with the edema around it, in elevated intracranial pressure (ICP). Elevated ICP is correlated with poor outcome, and may result in intubation, mechanical ventilation, multiple complications; if left untreated, it can be fatal. Thus, early diagnosis, monitoring and treatment are essential.
Two types of intracranial bleeding that cause hemorrhagic stroke are intracerebral hemorrhage and subarachnoid hemorrhage. Intracerebral or subarachnoid bleed can be accompanied by intraventricular hemorrhage (IVH), which is a bleeding into the brain’s ventricular system. IVH might occur after head trauma or as a result of hemorrhagic stroke. In this case as well, the volume of the bleeding is a predictive factor of prognosis and a valid method of evaluation is needed.
Automated brain hemorrhage segmentation
RSIP Vision’s image analysis algorithm module based on deep learning can rapidly estimate the hemorrhage volume and measure the edematous area around it for both ICH and IVH. Some cases of brain hemorrhage may require ICP monitoring and drainage via intra-ventricular catheter. Our image processing algorithms produce a 3D model of the ventricular system, which can ultimately be useful in guidance of the neurosurgeon during ventriculostomy.
SAH (subarachnoid hemorrhage) segmentation is challenging due to very variable and detailed shape, together with low contrast on CT. RSIP Vision used a large quantity of annotated data to feed a deep learning neural network able to segment all kinds of hemorrhages and accurately measure their volume. Cutting-edge Convolutional Neural Networks (CNN) architecture has been instrumental to achieve accurate results. Call our engineers to make sure that this module is what you are looking for.