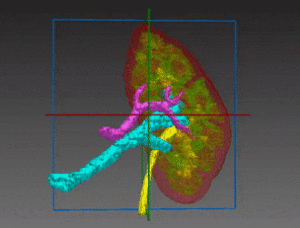
Kidney Segmentation
The most dramatically common kidney diseases are: kidney cancer, hitting 50,000 new patients every year only in the U.S.; and kidney failures, which leave the organ unable to remove wastes. Laparoscopic partial nephrectomy operations remove or reduce kidney tumors and some renal malfunctions. We at RSIP Vision help by providing a semi-automatic and very accurate kidney segmentation technique, built on deep learning and neural networks to create a kidney model which would be specific for each patient.
Read More
Pulmonary lobes segmentation
Emphysema quantification and lung nodule detection are among the clinical applications which benefit the most from lobes segmentation in CT scans. Proper lung segmentation is key to determine the boundaries of lobes and prevent pleural damage during examination and treatment. When correctly located, diseases are treated faster and better, hence the call for RSIP Vision to find a faster alternative to time-consuming manual segmentation.
Read More
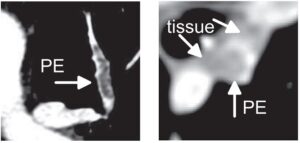
Pulmonary embolism detection
Timely detection of pulmonary embolism via CT angiography is key to reduce mortality risks. Human detection of pulmonary embolism (being often too slow, RSIP Vision recommends a combination of bidimensional and tridimensional image-processing techniques to achieve computer-aided pulmonary embolism detection which enables prompter diagnosis and treatment.
Read More
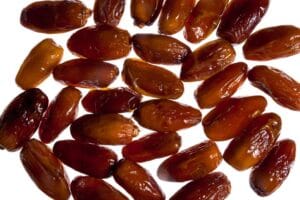
Date sorting
RSIP Vision has successfully worked in number of dates grading (or dates sorting) projects for our clients. Our automatic fruit recognition system is able to identify with high speed and accuracy all meaningful product features such as size, weight, defect, quality, color, texture, ripeness and others, offering key benefits to our clients: namely, fast and high-volume classification, savings in labor costs, consistent quality and reduced time-to-market.
Read More
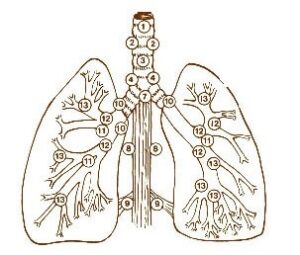
Lung Lymph Nodes Detection
Analyzing pulmonary lymph nodes can give us valuable information for lung cancer diagnosis and treatment. This solution too uses advanced algorithm of computer vision for pulmonology; it also allows to overcome technical difficulties like low image contrast and high nodes variation, offering a drastic improvement over techniques currently used to detect lung lymph nodes.
Read More
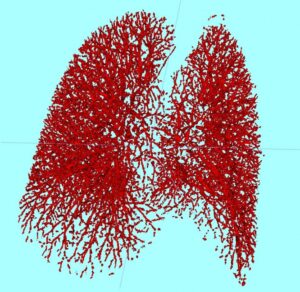
Lung vessel segmentation
Blood vessel segmentation of the lungs can help to identify important pulmonary diseases, characterizing nodules in the lungs, detecting pulmonary emboli and evaluating the lungs vasculature. Our technique of automatic pulmonary vessel segmentation completes very effectively the vessel tree structure provided by the CT scans of the lung, in such a way that the resulting image is more precise and matchlessly faster than any manual segmentation could be.
Read More
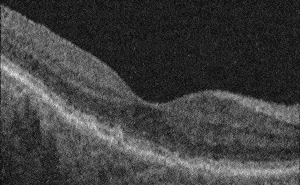
Measurement of Retinal Thickness
Retinal thickness is a key measurement used to assess the health of the retina and whether it needs any treatment. Thickness measures can be compared to optimal ranges or to data from the same patient over time, helping ophthalmologists to identify retinal disorders. Advanced optimization methods, borrowed from graph theory, enable us to solve the complex challenge of measuring retinal thickness within reasonable processing time.
Read More
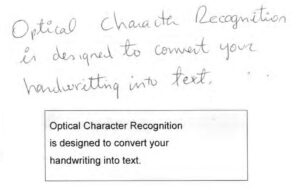
Deep Learning For OCR
OCR used for the visual inspection of documents has found wide application in both industry and research, though it is more commonly found in connection with printed characters than for handwritten ones, mainly owing to the variability in handwritten characters’ shapes and styles. Hence the need for automatic recognition performed by vision-based tailor-made algorithms and adjustment. Deep Neural Networks as a learning mechanism to perform recognition have proved to be particularly powerful tools, due to their high accuracy in both spotting text region and deciphering the characters.
Read More
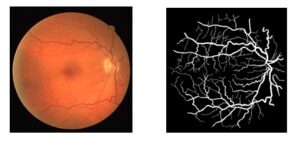
Vessel Segmentation Using Deep Learning
Various segmentation methods, whether based on Convolution Neural Networks or traditional image processing techniques, can be used to delineate the vascular tree in clinical imaging. Given the few features distinguishing veins from arteries (usually brighter and thinner than veins), the challenge consists of training a binary classifier assigning each pixel to the category of vein or artery. This article covers the advantages of using CNNs and deep neural networks for the classification and segmentation of vessels in fundus images.
Read More
Animal Monitoring With Pattern Recognition
Automatic Identification of Pigs in a Pen Using Pattern Recognition The growing demand for animal products is characterized, at the farmer’s end, by an