The localization and recognition of written characters has long been utilized by the industries for a variety of applications. Most Optical Character Recognition (OCR) system perform accurately when working in sterile environment, e.g. scanners and static scenes. With the advancement in technology, the demand for OCR in natural environment is on the rise. Most prominently, the localization and recognition of text outdoors, where conditions are far from being optimal for machine vision applications.
The challenges faced by machine-vision algorithms to localize and recognize text outdoors are numerous. Partial occlusion of written text, non-horizontal text orientation, non-uniform font style, blurring due to camera motion, and uneven illumination are only few examples of the dire conditions which are commonly found. Sharing the challenges of OCR, natural scene OCR needs also to group recognized characters into words. In addition, the more complex the scene is, the higher the computational burden. This latter aspect is a bottle-neck for the usability of cellphone based OCR and localization in natural scenes.
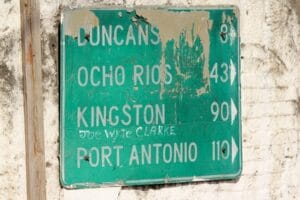
In the last decade, great progress has been made in the recognition of characters partially occluded and under heavy noise. A striking example is the ability of machine vision algorithms to perform almost equally well as humans in deciphering many of the Completely Automated Public Turing test to tell Computers and Humans Apart (CAPTCHA). Coupled with the advancement in natural scene analysis, the stage is set for utilization of OCR outdoors.
Real-time text localization and recognition is still a challenging task, with the golden standard reaching recognizing levels of about 70%. Conceptually, natural scene OCR is distinguished from traditional OCR by the step of text localization, which can be accounted for the relatively low recognition ratios. Scanning an image to localize text creates a target bank as potential text, though it is computationally intensive. To shorten the search time, probabilistic methods are utilized to account only for the most adequate text box found. In many cases, a ground-truth dictionary is scanned to give a probability measure for each character box in the scene.
Next, features from each box are extracted. These features describe the localized character’s properties, such as its perimeter, area, horizontal crossing and Euler number. Classifiers like neural-networks, adaBoost or random forests are then put into play by learning the features and adjusting their weights.
Since OCR in static equilibrated environment is progressive, the burden lies on the image processing part. To be able to put into action a real-time natural scene OCR, expertise in image analysis is thus imperative. RSIP Vision has been constructing advanced image processing algorithms for over 28 years. Many of RSIP Vision’s projects revolve around OCR. Visit our section on OCR projects to learn how RSIP Vision can help you with your real-time OCR challenge.
Real-time text localization and recognition is still a challenging task, with the golden standard reaching recognizing levels of about 70%. Conceptually, natural scene OCR is distinguished from traditional OCR by the step of text localization, which can be accounted for the relatively low recognition ratios. Scanning an image to localize text creates a target bank as potential text, though it is computationally intensive. To shorten the search time, probabilistic methods are utilized to account only for the most adequate text box found. In many cases, a ground-truth dictionary is scanned to give a probability measure for each character box in the scene.
Next, features from each box are extracted. These features describe the localized character’s properties, such as its perimeter, area, horizontal crossing and Euler number. Classifiers like neural-networks, adaBoost or random forests are then put into play by learning the features and adjusting their weights.
Since OCR in static equilibrated environment is progressive, the burden lies on the image processing part. To be able to put into action a real-time natural scene OCR, expertise in image analysis is thus imperative. RSIP Vision has been constructing advanced image processing algorithms for over 28 years. Many of RSIP Vision’s projects revolve around OCR. Visit our section on OCR projects to learn how RSIP Vision can help you with your real-time OCR challenge.